ARTIFICIAL INTELLIGENCE IMAGE GENERATION
Discover artificial intelligence image generation and how it transforms creativity and artistic expression!
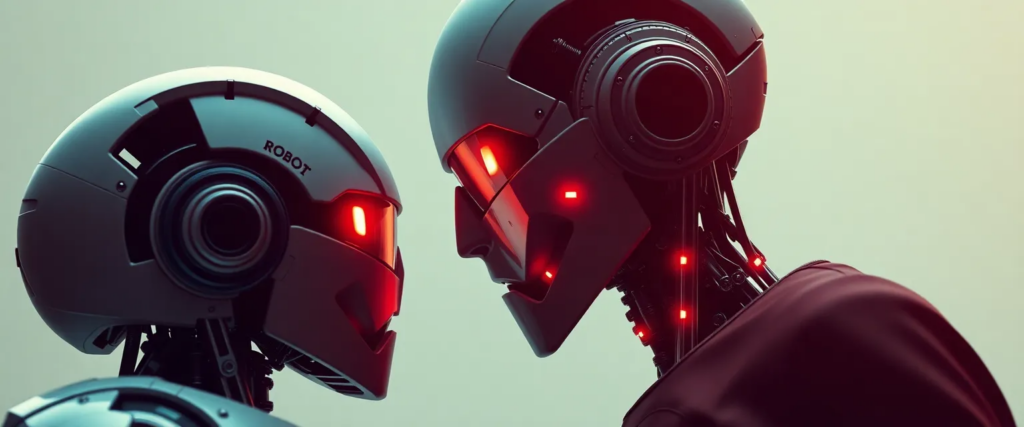
Unleashing Artificial Intelligence in Image Generation
The Evolution of AI in Image Creation – (https://nofiltergpt.ai)
Artificial intelligence has come a long way in making pictures. At first, AI was just a helper for simple image tweaks. But as time went on, machine learning and deep learning gave AI the power to whip up complex, high-quality images. This leap forward happened thanks to smarter algorithms and beefier computers.
It all started with basic programs that could mess around with existing pictures. As tech got better, more advanced models popped up, letting AI create brand-new images from scratch. Nowadays, AI can make art that gives human artists a run for their money, stretching the limits of visual creativity.
Year | Milestone in AI Image Generation |
---|---|
2014 | Generative Adversarial Networks (GANs) hit the scene |
2015 | First AI artwork goes under the hammer at auction |
2018 | Style Transfer techniques make their debut |
2021 | AI models start churning out photorealistic images |
Impact of AI on Artistic Expression
AI’s rise in image-making has shaken up the art world. Artists and creators now have AI tools to jazz up their work, try out new styles, and push creative limits. This team-up between human imagination and machine smarts has led to fresh art forms that were once just dreams.
AI-generated images can light a spark for artists, offering fresh ideas and angles. Plus, AI tools are now so easy to use that anyone can make eye-catching art, even without fancy training. This change has got folks talking about what creativity really means and how tech fits into making art.
But with AI in the mix, questions about who owns the art and what makes it original are popping up. As AI keeps getting better, it’s shaking up old ideas about what it means to be an artist. The chat about these shifts is ongoing, with many pondering what AI means for the future of art.
For more on what AI can do in image-making, check out our article on image generation ai models.
Understanding Artificial Intelligence Image Generation
Artificial intelligence image generation is where tech meets creativity, and it’s pretty mind-blowing. This section dives into how AI cooks up images and the cool ways this tech is being used.
How AI Generates Images
AI doesn’t just pull images out of thin air; it uses some serious brainpower. Here’s how it goes down:
- Data Collection: AI starts by hoarding a massive stash of images. Think of it as a buffet of styles, subjects, and formats.
- Training: The AI gets schooled using deep learning, picking up on patterns and features in the images. It’s like teaching a robot to see the world through our eyes, using neural networks that mimic how our brains work.
- Image Creation: Once the AI’s got its degree, it starts creating images by mixing and matching what it’s learned. This can lead to brand-new masterpieces or fresh takes on old favorites.
The magic of AI image generation hinges on the quality and variety of the training data. If you’re curious about the nitty-gritty of these models, check out our article on image generation ai models.
Applications of AI Image Generation
AI’s got its fingers in a lot of pies when it comes to image generation. Here are some standout uses:
Application | Description |
---|---|
Art Creation | AI can whip up original artwork, giving artists a new playground to mess around with styles and ideas. |
Advertising | Companies use AI-generated images to jazz up their marketing, cranking out eye-catching visuals in no time. |
Video Game Design | Game makers tap into AI to craft lifelike worlds and characters, making games more immersive. |
Fashion Design | Designers lean on AI to dream up clothing patterns and styles, making the design process a breeze. |
Film and Animation | AI-generated visuals spice up movie production, from concept art to jaw-dropping special effects. |
These examples show how AI is shaking things up across different fields, hinting at its power to transform creative work. As AI keeps getting smarter, its role in art and business is bound to grow. For more on how AI is changing the game, check out our article on uncensored ai technology.
Deep Learning in Image Generation
Deep learning is like the secret sauce in the world of AI image creation. It uses fancy algorithms and brainy networks to whip up images that look like they were crafted by a human artist. Let’s take a peek at how these neural networks work their magic in image creation and what it takes to train AI models to do this trick.
Neural Networks and Image Creation
Neural networks are the real MVPs in deep learning for image generation. Think of them as a web of neurons, much like the ones in our noggins, that chew through data. Each layer in this web picks out different bits and pieces from the input, helping the network learn and spit out images based on patterns it spots.
These networks come in all shapes and sizes, but when it comes to image generation, convolutional neural networks (CNNs) are the go-to. CNNs are champs at handling image tasks because they can catch the spatial hierarchies in pictures.
Layer Type | Function |
---|---|
Input Layer | Takes in the raw image data |
Convolutional Layer | Snags features from the image |
Activation Layer | Adds a twist with a non-linear function |
Pooling Layer | Shrinks the data while keeping the good stuff |
Output Layer | Pops out the final image |
Training AI Models for Image Generation
Training AI models to generate images is like teaching a dog new tricks. You feed them a ton of images, and they start to pick up on the styles and quirks of different pictures. Here’s how the training usually goes down:
- Data Collection: Rounding up a bunch of images to give the model a buffet of styles and subjects.
- Preprocessing: Tweaking and resizing images so they all play nice together.
- Model Training: Using algorithms to tweak the network’s weights based on the input. This often involves backpropagation, a fancy term for learning from mistakes.
- Evaluation: Checking how the model’s doing by making it generate images and seeing how they stack up against the originals.
- Fine-Tuning: Tweaking things to make the model sharper and more creative.
You can tell how well the training’s going by looking at metrics like loss and accuracy, which show how close the model is to hitting the mark.
Training Metric | Description |
---|---|
Loss | Shows the gap between the generated image and the target image |
Accuracy | Tells you the percentage of images that hit the bullseye |
Getting a handle on neural networks and the training process is key to understanding what AI can do in image generation. For more juicy details on the models used in this field, check out our article on image generation ai models.
Exploring AI Image Generation Techniques
Artificial intelligence is shaking up the art scene with some mind-blowing image generation tricks. Let’s check out three big players in this game: Generative Adversarial Networks (GANs), Variational Autoencoders (VAEs), and Style Transfer.
Generative Adversarial Networks (GANs)
Generative Adversarial Networks, or GANs, are like the rock stars of AI image creation. They work with two neural networks: the generator and the discriminator. The generator’s job is to whip up images, while the discriminator plays the critic, deciding if they’re the real deal or not. This back-and-forth continues until the generator nails it, making images that look just like the real thing.
Component | Function |
---|---|
Generator | Whips up new images from scratch |
Discriminator | Judges images and gives feedback |
GANs are the go-to for everything from creating art to designing video games and even fashion. Their knack for producing top-notch images has made them a hit with artists and developers. Want to dive deeper into AI models? Check out our piece on image generation ai models.
Variational Autoencoders (VAEs)
Variational Autoencoders, or VAEs, are another cool tool in the AI image-making kit. They take an image, squish it down into a compact form, and then rebuild it. This lets VAEs get a feel for the data’s vibe, so they can churn out new images that echo the originals.
Feature | Description |
---|---|
Encoder | Squishes images into a compact form |
Decoder | Rebuilds images from the compact form |
VAEs are great for tweaking existing images, making them a favorite in design and creative fields. They offer a fresh way to play with image generation while keeping a nod to the original stuff.
Style Transfer in AI Image Generation
Style Transfer is where things get artsy. It lets you mix two images: one for the content and another for the style. Using deep learning, it slaps the artistic flair of one image onto the content of another, creating something totally new.
Process | Description |
---|---|
Content Image | The image that keeps its content |
Style Image | The image that lends its artistic flair |
Style Transfer is a hit among artists, letting them mash up different styles to create new masterpieces. It shows off AI’s flexibility in image generation and its power to spark creativity.
These techniques are just the tip of the iceberg in AI image generation. As tech keeps pushing forward, the ways we can create art and visuals will only grow, opening up new paths for creativity. Curious about the bigger picture of AI? Check out our article on uncensored ai technology.
Ethical Considerations in AI Image Generation
As AI keeps cranking out images, the ethical side of things is getting more attention. Tackling bias and using AI responsibly are key to making sure digital art is fair and welcoming to everyone.
Addressing Bias in AI-Generated Images
Bias in AI images often comes from the data used to train the models. If the data is narrow-minded or full of stereotypes, the images might end up reflecting those biases. This can lead to reinforcing harmful stereotypes and misrepresenting certain groups.
To fight bias, developers need to focus on diverse datasets that truly represent different cultures, genders, and backgrounds. Regular check-ups on AI models can help spot and fix biases in image generation. Here’s a quick look at where bias in AI images usually comes from:
Source of Bias | Description |
---|---|
Training Data | Skewed results from limited or biased datasets. |
Algorithm Design | Decisions during model creation can introduce bias. |
User Input | Biased prompts or instructions can lead to biased outputs. |
Ensuring Responsible Use of AI in Image Creation
Using AI responsibly in image creation means following ethical guidelines and best practices. Artists, developers, and users need to be aware of the potential fallout from their creations. This includes understanding how AI-generated images might be used in advertising, media, and social platforms.
Setting clear rules for using AI-generated content can help avoid misuse. This means respecting copyright laws, steering clear of harmful or misleading images, and being upfront about using AI in artistic processes. Here’s a rundown of key principles for responsible AI image generation:
Principle | Description |
---|---|
Transparency | Always let folks know when images are AI-generated. |
Accountability | Creators should own up to the content they produce. |
Inclusivity | Aim for diverse representation in AI-generated images. |
By tackling bias and encouraging responsible practices, AI image generation can flourish while keeping ethical issues in check. For more on the impact of AI tech, check out our article on uncensored ai technology.
Future Trends in AI Image Generation
Advancements in AI Technology
AI image generation is on a fast track to becoming more impressive by the day. With tech getting smarter, we’re seeing algorithms and models that churn out images with better quality and creativity. Deep learning and neural networks are getting a makeover, making the images they produce look more lifelike and varied.
A big deal in this space is the use of unsupervised learning, where AI picks up skills from data that hasn’t been labeled. This gives it more room to be creative. Plus, with beefed-up hardware like GPUs and TPUs, things are speeding up, letting us handle bigger piles of data without breaking a sweat.
Advancement | Description |
---|---|
Unsupervised Learning | AI learns from unlabelled data, boosting creativity. |
Improved Algorithms | Smarter models make better images. |
Enhanced Hardware | Faster processing with advanced GPUs and TPUs. |
Potential Impact on the Art Industry
AI image generation is shaking things up in the art world. Artists are starting to see AI as a buddy, using it to spark new ideas and stretch the limits of what art can be. This team-up can lead to fresh, groundbreaking pieces that mix human flair with machine magic.
But, there’s a catch. As AI gets more involved in art, questions pop up about who really owns the work and what makes it original. As AI-generated art becomes more common, artists might have to rethink their methods and figure out how to weave AI into their creative flow.
Impact | Description |
---|---|
Collaboration | Artists use AI for inspiration and creativity. |
Redefining Art | Ideas of authorship and originality might shift. |
New Opportunities | AI paves the way for new art forms and expressions. |
The future of AI image generation is buzzing with potential. As tech keeps pushing forward, the bond between AI and art is set to grow, sparking new ways to express and create. For more on how AI is changing the game, check out our article on uncensored ai technology.
Challenges and Limitations of AI Image Generation
AI image generation is like a rollercoaster ride—exciting but with its ups and downs. As it keeps growing, it bumps into some hurdles that affect how well it works and how folks feel about it. Let’s dive into the quirks of AI art and the tug-of-war between creativity and automation.
Uncertainties in AI-Generated Art
AI art can be a bit of a head-scratcher. Is it really original? Since AI learns from existing stuff, there’s a big question mark over how unique its creations are. Some artists and critics think AI misses the emotional punch and personal touch that humans bring to the canvas. This skepticism can make people wonder if AI art is worth its salt in the art world.
Aspect | Description |
---|---|
Authenticity | Is AI art truly original, or just a remix of what’s already out there? |
Emotional Depth | Can AI really tug at your heartstrings like a human artist? |
Value Perception | Is AI art as valuable as the good old traditional stuff? |
Bias is another sticky issue. If AI learns from skewed data, it might churn out biased images, raising ethical eyebrows. Curious about this? Check out our piece on uncensored ai technology.
Balancing Creativity and Automation
AI in image-making is a bit of a balancing act. Sure, it can whip up images in a flash, but there’s a risk it might put a damper on human creativity. Artists might lean too much on AI, leading to cookie-cutter styles and less room for fresh ideas.
Factor | Impact |
---|---|
Speed | AI’s quick output might overshadow the creative journey. |
Homogenization | Too much AI reliance could make art look samey. |
Innovation | Keeping art fresh and unique in an AI-driven world is a real challenge. |
Striking the right balance between using AI for speed and keeping human creativity alive is key. Artists and tech whizzes need to team up to make sure AI is a helper, not a replacement. For more on what AI can do, have a look at our article on image generation ai models.
Leave a Reply