Unleash Your Creative Vision with CreatorFrames.com: The Ultimate AI-Powered Platform for NSFW and All Content Animation
Welcome to CreatorFrames.com, the cutting-edge platform revolutionizing content creation with AI-driven video generation. Whether you’re crafting bold NSFW content or exploring a wide range of creative ideas, our beta testing platform empowers creators to bring their visions to life with unmatched precision and flexibility. By harnessing the power of advanced AI models like Wan 2.1 and Hunyuan, CreatorFrames.com offers a seamless, high-quality experience for animating everything from realistic scenes to stylized animations. Here’s why our platform is your go-to destination for limitless creative expression.
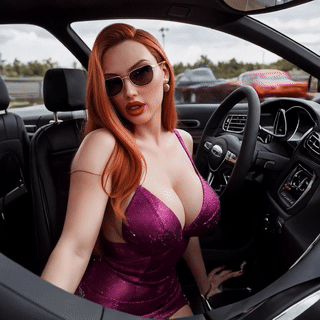
From this:
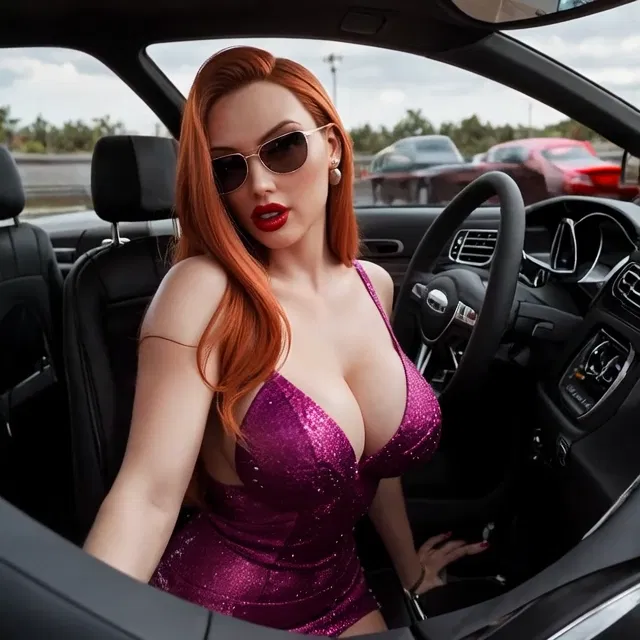
Why Choose CreatorFrames.com?
At CreatorFrames.com, we believe in giving creators the freedom to explore their ideas without boundaries. Our platform is designed to support a diverse range of content, including NSFW, with tools that prioritize accuracy, quality, and ease of use. Whether you’re a professional animator, a content creator, or a hobbyist, our AI-driven workflows make it simple to produce stunning videos that align perfectly with your creative intent.
Key Features of CreatorFrames.com:
- Unrestricted Content Creation: Unlike many platforms with heavy restrictions, CreatorFrames.com embraces creative freedom. Create NSFW content, realistic visuals, anime-style animations, or anything in between—our platform supports it all.
- Powered by Wan 2.1 and Hunyuan: Our integration with state-of-the-art AI models like Wan 2.1 and Hunyuan ensures exceptional video quality, realistic motion, and precise prompt adherence.
- User-Friendly Interface: No advanced technical skills? No problem. Our intuitive interface and pre-configured workflows make video generation accessible to creators of all levels.
- High-Quality Output: Generate videos with sharp visuals, smooth animations, and cinematic flair, thanks to the advanced architectures of Wan 2.1 and Hunyuan, featuring billions of parameters for unparalleled fidelity.
- Beta Testing Perks: As a beta tester, you’ll get early access to cutting-edge features, exclusive updates, and the chance to shape the future of CreatorFrames.com.
Wan 2.1: Precision and Realism at Its Best
Wan 2.1, developed by Alibaba Cloud, is a powerhouse in AI video generation, excelling in creating high-quality visuals with complex motion and realistic details. Its proprietary Variational Autoencoder (VAE) and Denoising Diffusion Transformer (DiT) architecture ensure frame-to-frame consistency, making it ideal for animating intricate scenes like dynamic character interactions or NSFW content with lifelike precision.
Why Wan 2.1 Stands Out:
- Prompt Adherence: Wan 2.1 follows text prompts with remarkable accuracy, ensuring your vision translates seamlessly into video. Whether you’re animating a hyper-realistic scene or a stylized NSFW clip, Wan 2.1 delivers.
- Realistic Motion: From figure skating to intimate character interactions, Wan 2.1 handles large-scale movements and subtle nuances with ease, maintaining body coordination and natural trajectories.
- Versatility: Supports both English and Chinese prompts, making it accessible for global creators crafting diverse content types.
In beta testing on CreatorFrames.com, Wan 2.1 has been praised for its ability to generate smooth, high-fidelity videos, especially for character-driven animations and NSFW content. Users have noted its superior performance in maintaining visual sharpness and prompt coherence compared to other models.


Hunyuan: A Game-Changer for Stylized and NSFW Content
Tencent’s Hunyuan Video model, with its 13 billion parameters, is another cornerstone of CreatorFrames.com. Known for its versatility, Hunyuan excels in both realistic and stylized outputs, making it a favorite for creators exploring anime-style animations or NSFW content. Its Multimodal Large Language Model (MLLM) ensures precise interpretation of detailed prompts, delivering videos that capture your creative intent with stunning accuracy.


Hunyuan’s Strengths:
- Flexible Styling: Hunyuan shines in generating stylized content, such as anime or cartoon-like visuals, while also handling realistic scenes with ease. Its ability to switch seamlessly between styles makes it perfect for diverse projects.
- NSFW Capabilities: Hunyuan’s uncensored nature allows creators to explore NSFW content without restrictive filters, supported by fine-tuned LoRAs for enhanced quality and identity preservation.
- Efficient Processing: Hunyuan delivers high-quality results with lower computational demands, making it accessible for creators with mid-range hardware.
Hunyuan’s integration with ComfyUI on CreatorFrames.com simplifies the creation process, offering pre-configured workflows that minimize setup time and maximize creative output. Whether you’re animating a single character or complex multi-person scenes, Hunyuan’s advanced understanding of prompts ensures professional-grade results.

Crafting NSFW Content with Confidence
CreatorFrames.com is designed to empower creators to explore NSFW content responsibly and creatively. Both Wan 2.1 and Hunyuan offer robust capabilities for generating high-quality NSFW videos, with features like:
- LoRA Support: Fine-tune models with LoRAs to enhance specific styles or identities, ensuring consistent and detailed NSFW outputs.
- Image-to-Video (I2V): Start with a single image and animate it into a dynamic NSFW video, with Wan 2.1 excelling in motion consistency and Hunyuan offering superior detail in stylized content.
- Community Insights: Our beta testing community actively shares workflows and tips for optimizing NSFW content, ensuring you can achieve the best results with minimal trial and error.
While NSFW content creation is fully supported, we encourage responsible use in line with applicable laws and platform guidelines. Our beta testing phase allows you to experiment with these tools in a safe, creator-focused environment.
How to Get Started with CreatorFrames.com
Ready to dive into the world of AI-powered video creation? Joining the CreatorFrames.com beta is simple:
- Sign Up: Register for free on CreatorFrames.com to access our beta testing program.
- Choose Your Model: Select Wan 2.1 for realistic, high-fidelity animations or Hunyuan for stylized and NSFW content.
- Craft Your Prompt: Use our prompt engineering guide to create detailed, precise instructions for the AI. Include specifics like scene, motion, lighting, and style for optimal results.
- Generate and Refine: Use our intuitive interface to generate videos, tweak settings, and experiment with LoRAs for custom outputs.
- Share Feedback: As a beta tester, your input helps shape the platform. Share your creations and suggestions to help us improve!
Why Beta Test with CreatorFrames.com?
By joining our beta program, you’re not just testing a platform—you’re helping redefine the future of AI-driven content creation. Here’s what you gain:
- Early Access: Be among the first to explore Wan 2.1 and Hunyuan’s latest features.
- Community Collaboration: Join a vibrant community of creators sharing workflows, tips, and NSFW content strategies.
- Shape the Platform: Your feedback directly influences CreatorFrames.com’s development, ensuring it meets the needs of creators like you.
The Future of Content Creation is Here
CreatorFrames.com is more than a platform—it’s a creative playground where your imagination knows no bounds. Whether you’re animating NSFW content, crafting cinematic masterpieces, or experimenting with anime-style visuals, our integration of Wan 2.1 and Hunyuan delivers unparalleled accuracy and quality. Join our beta testing community today and experience the future of AI-powered video creation.
Get Started Now: Visit CreatorFrames.com to sign up for the beta and start creating stunning, AI-generated videos. Your creative journey begins here!
Note: Always ensure compliance with local laws and platform guidelines when creating NSFW content. For more details on Wan 2.1, visit Alibaba Cloud’s official site, and for Hunyuan, check out Tencent’s HunyuanVideo page.

